Miles Cranmer是剑桥大学助理教授,他于2024年4月在Simons Foundation发表的演讲The Next Great Scientific Theory is Hiding Inside a Neural Network很有启发性。
俺对视频内容的总结:先“形而下”,再“形而上”。先把“模式”压缩进AI模型,再用“符号蒸馏”探究“模式”后面的理论:1)如为已知理论➡️可解释AI(XAI);2)如为未知规律➡️可能是理论新发现。
Summary for the video: first, compress the “patterns” into the AI model, and then use “symbolic distillation” to explore the theory behind the “patterns”: 1) If the theory is known ➡️ explainable AI (XAI); 2) If the theory is unknown ➡️ it may be a new theoretical discovery.
Google Gemini对视频的总结:
Miles Cranmer 在演讲中提出,解释神经网络可以成为发现科学新知的新方法。 他提出了一种名为符号蒸馏的方法来实现这一目标。
传统的科学方法是建立理论来描述数据。 Cranmer 认为,随着强大神经网络的兴起,一种新的方法成为可能。 神经网络可以训练大量数据,并发现现有理论中未包含的模式。 挑战在于从神经网络中提取这些见解并将它们纳入科学理解。
Cranmer 提出符号蒸馏作为实现这一目标的方法。 该方法涉及通过找到一组复制网络行为的方程来解释神经网络。 然后可以分析这些方程来理解网络学习的底层科学原理。
Cranmer 承认符号蒸馏存在局限性,特别是它无法找到复杂的符号模型。 但是,他认为这是科学发现的一个令人兴奋的方向,尤其是随着像 Polymathic AI 这样的基础模型的发展。 这些模型在多个学科上训练了大量数据集,并且可能包含广泛应用的科学模型。
演讲以对开放问题的讨论结束。 一个问题是如何将更一般的算法(超出简单的数学方程)纳入符号回归。 另一个问题是,预先训练神经网络处理某些类型的数据是否会对科学发现造成不利影响。 Cranmer 承认可能存在对抗性示例,其中预先训练某些数据集可能会使发现科学见解更加困难,但迄今为止尚未发现此类示例。
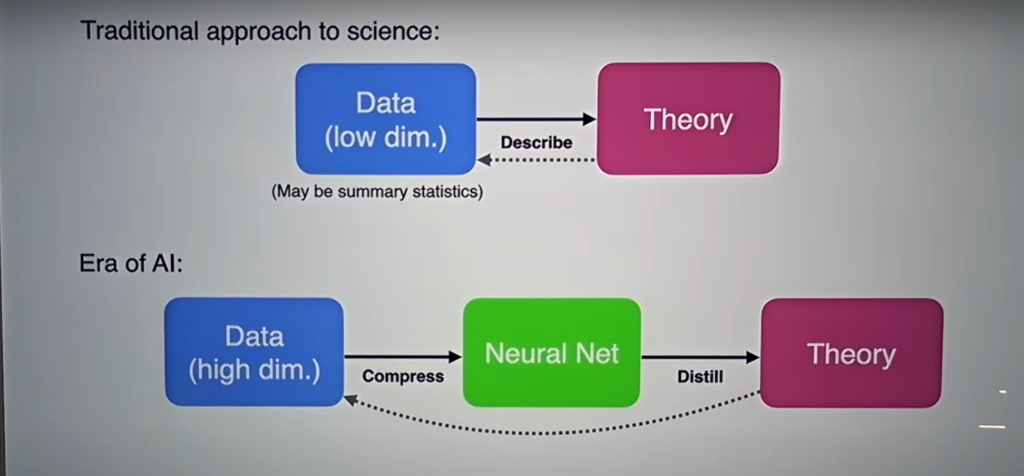
Video summary by Google Gemini:
This talk by Miles Cranmer argues that interpreting neural networks can be a new way to discover scientific insights. Cranmer proposes a method called symbolic distillation to achieve this.
The traditional scientific approach involves building theories to describe data. Cranmer argues that with the rise of powerful neural networks, a new approach is possible. Neural networks can be trained on massive amounts of data and find patterns that are not included in existing theories. The challenge is to distill these insights from the neural networks and incorporate them into scientific understanding.
Cranmer proposes symbolic distillation as a method to achieve this. This method involves interpreting a neural network by finding a set of equations that replicates the network’s behavior. These equations can then be analyzed to understand the underlying scientific principles learned by the network.
Cranmer acknowledges that there are limitations to symbolic distillation, particularly its inability to find complex symbolic models. However, he believes that this is an exciting direction for scientific discovery, especially with the development of foundation models like Polymathic AI. These models are trained on massive datasets across many disciplines and are likely to contain broadly applicable scientific models.
The talk concludes with a discussion of open questions. One question is how to incorporate more general algorithms, beyond simple mathematical equations, into symbolic regression. Another question is whether pre-training neural networks on certain types of data can be detrimental to scientific discovery. Cranmer acknowledges that there are likely to be adversarial examples, where pre-training on certain data sets can make it harder to find scientific insights, but so far such examples haven’t been discovered.