很不幸,假消息(或者说错误消息)就是比真消息传播得更远、更快、更深、更广。
2018年3月,MIT的三位学者Soroush Vosoughi,Deb Roy,Sinan Aral在Science杂志上发表了研究论文,The spread of true and false news online,揭示了真假消息传播的一些事实真相。
主要的研究结论如下:
1.Falsehood diffused significantly farther, faster, deeper, and more broadly than the truth in all categories of information, and the effects were more pronounced for false political news than for false news about terrorism, natural disasters, science, urban legends, or financial information.
虚假传播扩散明显要比真相传播更远、更快、更深、更广,其中政治相关的假消息尤甚。
2.We found that false news was more novel than true news, which suggests that people were more likely to share novel information.
假消息的新奇(新鲜)度往往高于真消息,这也意味着人们喜欢分享新奇(新鲜)的消息。
3.Whereas false stories inspired fear, disgust, and surprise in replies, true stories inspired anticipation, sadness, joy, and trust.
假消息往往带给人恐惧、厌恶和惊讶,而真消息引起期待、伤心、快乐和信任。
4.Contrary to conventional wisdom, robots accelerated the spread of true and false news at the same rate, implying that false news spreads more than the truth because humans, not robots, are more likely to spread it.
人们常以为机器人账号会加剧假消息的传播,但事实是机器人账号同等加速真、假消息的传播,这意味着假消息的传播,人为因素是关键。
研究的数据样本来自Twitter,数据是2006年到2017年间被传播的约126,000条消息,这些消息被约3百万人传播了超过450万次。应该说研究的数据样本足够典型和足够大。
论文中的图表直观展示了真、假消息传播的对比,例如下面这些图:
图中涉及的一些基本概念如下:
1.CCDF, Complementary Cumulative Distribution Function.
互补累积分布函数:对连续函数,所有大于a的值,其出现概率的和。举例:所有被研究的传播链中,传播链深度大于10的传播链出现概率的和。
2.Cascade Depth, the number of retweet hops from the origin tweet over time, where a hop is a retweet by a new unique user.
传播链深度: 消息被单一不同用户转发的层级数。
3.Cascade Size, the number of users involved in the cascade over time.
传播链规模:消息传播涉及的所有用户的数量。
4.Maximum Breadth, the maximum number of users involved in the cascade at any depth.
最大传播宽度:消息被转发过程中,任一转发层级的最大用户数。
5.Structural Virality, a measure that interpolates between content spread through a single, large broadcast and that which spreads through multiple generations, with any one individual directly responsible for only a fraction of the total spread.
结构性传播度:姑且这么翻译。理解下来应该是一个标度值,该标度值在“传播次数少但广”与“传播次数多但每次涉及的用户较少”之间取值。本论文的取值范围为1-100,数值越大,穿透力越强。
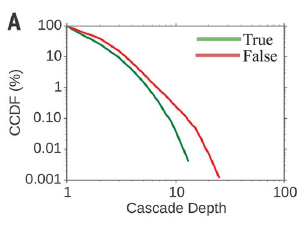
2.相同消息数量,例如CCDF为0.01%时,假消息的传播链深度超过19,比真消息高出约8。
——
Source: The spread of true and false news online, Soroush Vosoughi,Deb Roy,Sinan Aral
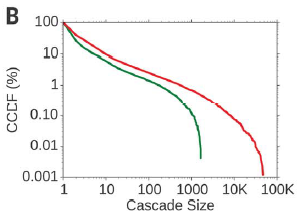
2.真消息传播范围超过1000人都较难,但1%的假消息的传播范围则在1000-100000人之间。
——
Source: The spread of true and false news online, Soroush Vosoughi,Deb Roy,Sinan Aral
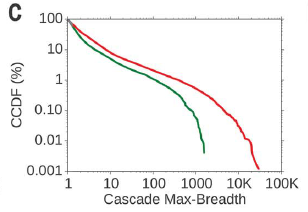
——
Source: The spread of true and false news online, Soroush Vosoughi,Deb Roy,Sinan Aral
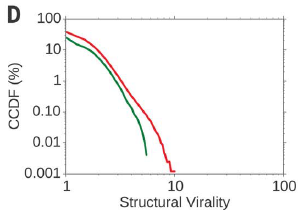
——
Source: The spread of true and false news online, Soroush Vosoughi,Deb Roy,Sinan Aral
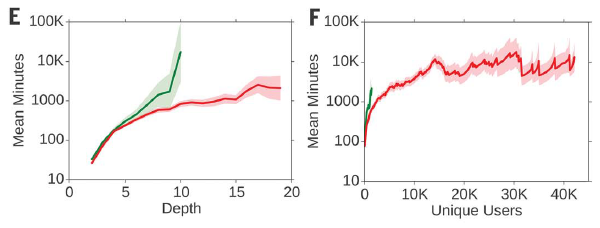
2.真消息的传播链深度要达到10,需要的时间是假消息传播的20倍;
3.真消息的传播链深度很难超过10;
4.假消息传播链深度达到19的速度,要比真消息传播链深度达到10的速度快10倍。
Source: The spread of true and false news online, Soroush Vosoughi,Deb Roy,Sinan Aral
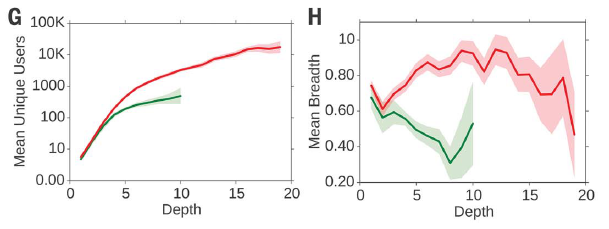
2.假消息的扩散广度明显高于真消息。
Source: The spread of true and false news online, Soroush Vosoughi,Deb Roy,Sinan Aral
上述图文说明,只是对论文内容的少量摘录。欲了解论文全部内容,可以下载其PDF版。
论文结尾提到,由于信息误导更多是人的行为使然,故限制虚假消息及其传播的对策重点不应该是消除社交平台的机器人账号,而应该是对人的行为的干预,比如对自觉规避信息误导或者阻止信息误导的行为进行激励。
干预行为当然也包括封号(论文没这么说),例如今年1月份特朗普的Twitter账号被封。说不准,Twitter采取封号措施的决策依据之一,就是这篇论文的研究结论—假消息的传播力远远高于真消息。
“转发消息之前,略微想一想”(原话“Think before you retweet.”),这是来自论文作者之一Roy的提醒。