向ChatGPT 4o问了关于Neural Operator的问题:神经算子(Neural operators)是专业领域AI泛化(Generalization)的方向?
我觉得其回答还是蛮专业,蛮有参考价值的。
神经算子(Neural Operators)代表了专业领域内AI泛化的一项重要进展,尤其是在解决偏微分方程(PDE)支配的复杂问题时。这些算子旨在学习函数空间之间的映射,使其能够在不同问题实例之间进行泛化,这比传统神经网络通常专注于固定维度的输入输出映射更进一步。
主要优势:
- 多功能性:神经算子能够处理各种问题,而无需为每个特定实例进行重新训练。这使得它们在流体动力学、天气预报等PDE普遍存在的应用领域非常多功能。
- 效率:与传统的PDE数值求解方法相比,神经算子通常更具计算效率。一旦训练完成,神经算子可以快速提供解决方案,使其适用于实时应用。
- 可扩展性:神经算子有可能扩展到高维问题,这对传统方法来说是一个挑战。这种可扩展性为解决科学和工程领域中以前难以处理的问题开辟了新可能性。
- 可迁移性:它们表现出跨不同领域转移知识的潜力。例如,在一种物理系统上训练的神经算子可以通过最少的额外训练适应另一种系统,利用系统之间的基本相似性。
挑战与考虑:
- 训练数据:高质量、多样化的训练数据对于神经算子的良好泛化至关重要。收集和整理这些数据可能是一个重大挑战。
- 可解释性:像许多深度学习模型一样,神经算子可能被视为黑箱,这对于需要高可解释性和可信度的应用来说可能是个问题。
- 理论理解:为什么神经算子表现如此优秀的理论基础仍在探索中。更深入的理解对于进一步改进和优化这些模型是必要的。
- 计算资源:训练神经算子可能需要大量计算资源,尤其是对于高维问题。
应用与未来方向:
神经算子在气候建模、天气预测、材料科学及涉及复杂物理模拟的任何领域都非常有前景。未来的研究可能会集中在提高这些模型的效率和准确性,发展更好的理论基础,并探索各种科学和工程领域的新应用。
总之,神经算子在推进专业领域内AI泛化方面具有巨大潜力,提供了一种更高效和适应性更强的工具来解决复杂问题。然而,它们的成功实施将取决于克服数据、可解释性、理论理解和计算需求方面的挑战。
Neural operators represent a significant advancement in the generalization of AI within specialized fields, particularly for solving complex problems governed by partial differential equations (PDEs). These operators aim to learn mappings between function spaces, allowing them to generalize across different instances of problems, which is a step beyond traditional neural networks that typically focus on fixed-dimensional input-output mappings.
Key Advantages:
- Versatility: Neural operators can handle a wide range of problems without the need for retraining for each specific instance. This makes them highly versatile for applications in fluid dynamics, weather forecasting, and other areas where PDEs are prevalent.
- Efficiency: They are often more computationally efficient compared to traditional numerical methods for solving PDEs. Once trained, neural operators can provide solutions quickly, making them suitable for real-time applications.
- Scalability: Neural operators can potentially scale to high-dimensional problems, which is challenging for conventional methods. This scalability opens up new possibilities for solving previously intractable problems in science and engineering.
- Transferability: They exhibit the potential to transfer knowledge across different domains. For example, a neural operator trained on one type of physical system could be adapted to another with minimal additional training, leveraging the underlying similarities between the systems.
Challenges and Considerations:
- Training Data: High-quality, diverse training data is essential for neural operators to generalize well. Collecting and curating such data can be a significant challenge.
- Interpretability: Like many deep learning models, neural operators can be seen as black boxes, which might be problematic for applications requiring high interpretability and trustworthiness.
- Theoretical Understanding: The theoretical underpinnings of why neural operators work so well are still being explored. A deeper understanding is necessary to further refine and optimize these models.
- Computational Resources: Training neural operators can be resource-intensive, requiring substantial computational power, especially for high-dimensional problems.
Applications and Future Directions:
Neural operators are particularly promising in fields like climate modeling, weather prediction, material science, and any area involving complex physical simulations. Future research will likely focus on improving the efficiency and accuracy of these models, developing better theoretical foundations, and exploring new applications across various scientific and engineering domains.
In summary, neural operators hold great promise for advancing AI generalization in specialized fields, offering a powerful tool for tackling complex problems with greater efficiency and adaptability. However, their successful implementation will depend on overcoming challenges related to data, interpretability, theoretical understanding, and computational demands.
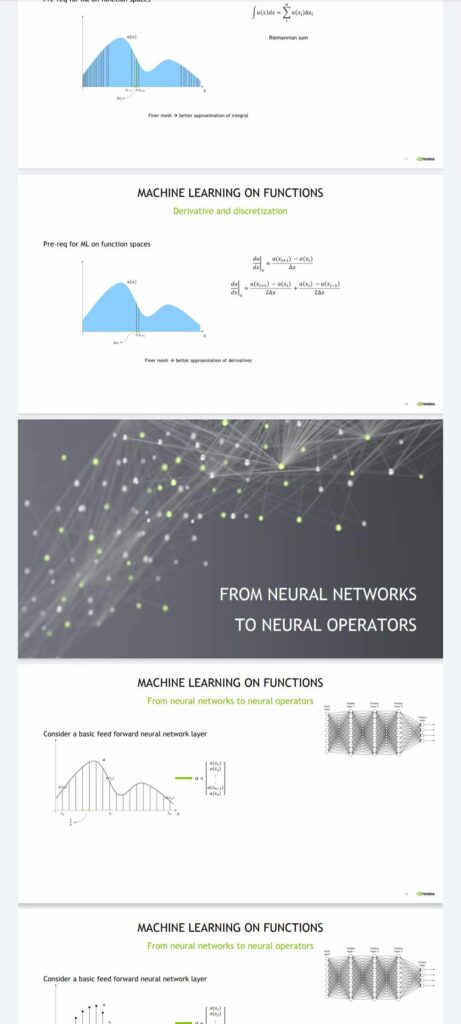
#NEURAL OPERATORS
Kamyar Azizzadenesheli