1、边缘端AI模型的部署将会越来越容易。越来越多的大厂,如芯片和工业自动化大厂,已经或将会推出自己的边缘设备AI模型生成、处理和部署工具;
2、对于被监测的设备或需要处理的对象,重要的是根据需求确定能够体现其结构化特征的表征监测项。同样重要的是,如何采集表征监测项数据;
3、更重要的是用于AI模型学习的数据,包括数据的获取和必要的标识;
4、关键问题的关键样本数据反而不容易获取。小样本学习是途径之一,合成数据也是一个方向;
5、如果能够用数据分析的数学模型表述出设备或对象的内在结构化特征,就不一定要用AI模型。如果面对的是个黑盒,那用AI模型的锤子先砸一砸也无妨。
1. The deployment of edge AI models is becoming increasingly easier. More and more major companies, such as chip manufacturers and industrial automation giants, have launched or will launch their own tools for generating, processing and deploying edge AI models.
2. For the monitored devices or objects that need processing, it is crucial to determine the representative monitoring items that can reflect their structured characteristics based on the requirements. Equally important is how to collect the data for these representative monitoring items.
3. Even more important is the data used for AI model training, including the acquisition and necessary labeling of the data. In a sense, better data is better than better models.
4. Key sample data for critical issues are rather difficult to obtain. Few-shot learning is one of the approaches. Synthetic data is another direction.
5. If the intrinsic structured characteristics of the device or object can be described using mathematical models for data analysis, there may not be a need for AI models. However, if faced with a black box, it’s acceptable to first try using the AI model approach.
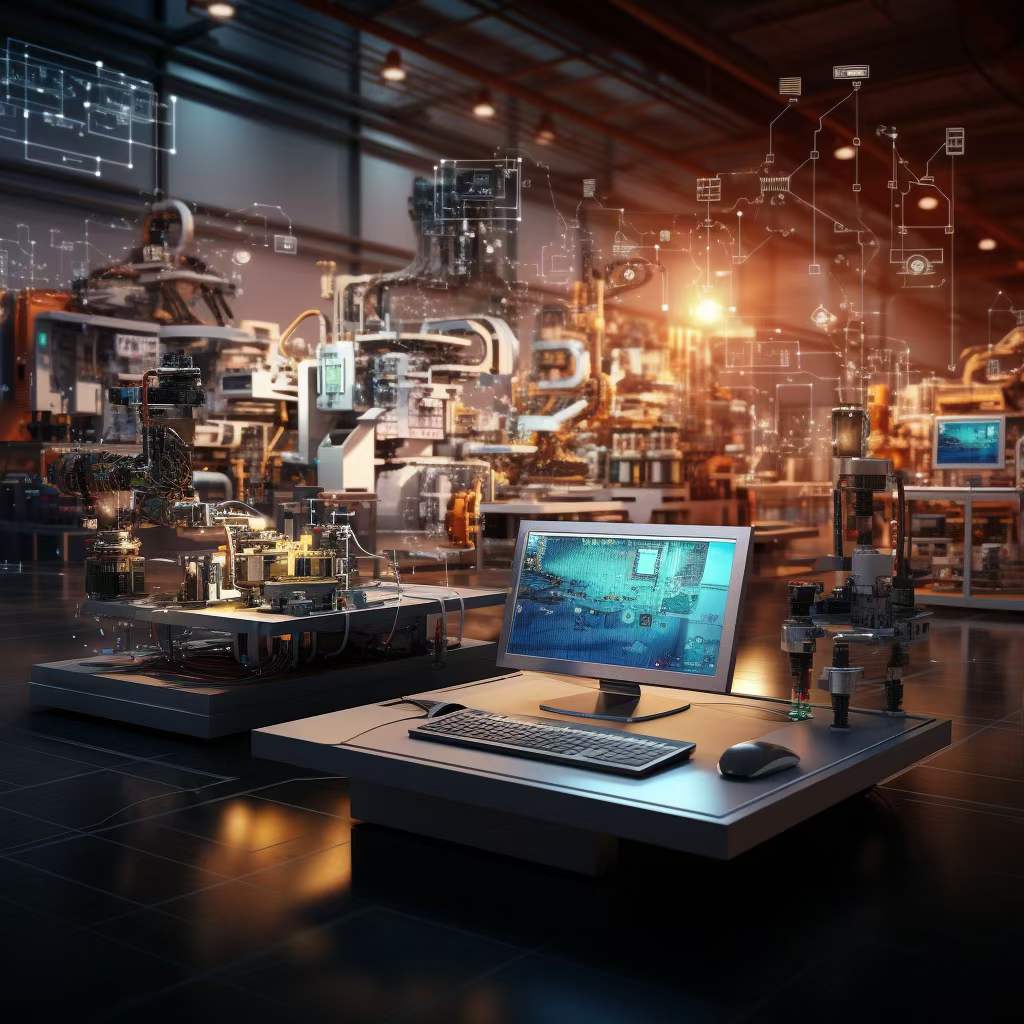